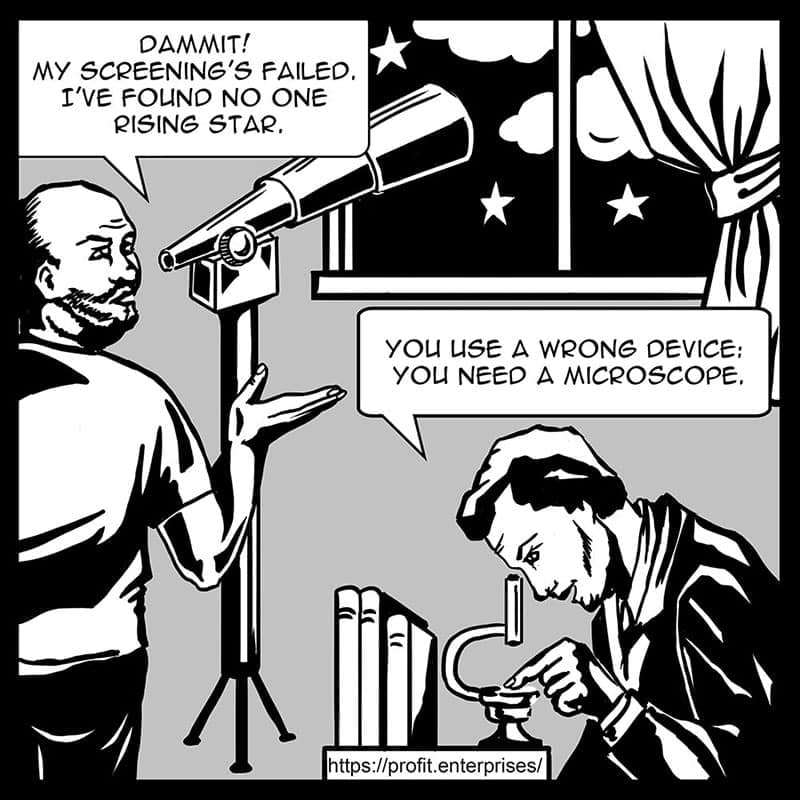
Until recently, startup sourcing and screening was a manual process, more art than science. Investors played it by ear, relying heavily upon their own set of qualitative metrics and “gut feeling”. Nevertheless, the quantitative data-driven approach is coming, and Artificial Intelligence/Machine Learning (AI/ML) algorithms help to collect raw data from different sources and process it into meaningful information. However, a quantitate approach meets serious challenges and needs a data framework to be practical. The PROFIT.enterprise platform will change the whole process dramatically, engaging human intellect to harness proprietary startup information for further algorithmic processing with active investors’ engagement.
Building a Knowledge Pyramid
Screening early-stage startups to find promising ones, investors have to deal with data. The process is influenced by three trends. First, there is startup proliferation with the pace of one new startup per day. Second, the PE world is changing, and traditional investors’ patterns and frameworks they used to discover promising business opportunities do not work now. Third, the industry focuses today on intangible assets, knowledge-based and lacking a physical form. Nevertheless, “In a nutshell, the VC investment/decision-making process is manual, inefficient, non-inclusive, subjective and biased [1]).
It would be wrong to think that the abundance of a startup looking for funding is a boon. The situation is quite the reverse. Struggling with a stream of low-quality data, investors waste their time screening startups that are unworthy of attention. The process takes about 20% of investors’ time with more than modest outcomes, taking into consideration that a typical deal takes 83 days to close, with 118 hours for due diligence. Out of 100 selected startups:
- 28 lead to the first meeting.
- 10 is reviewed by partners.
- 4 proceed to due diligence.
- 1 is selected for funding. [2]
Processing manually big volumes of unstructured and often unreliable data, an investor not only searches in irrelevant data sets but also cannot recognise and lose some good business opportunities. Today’s SS process is characterised by information asymmetry. Early-stage investors have to make funding decisions using substantially incomplete data about startups, and they lack effective methods to process this data into insight-generating information. On the other hand, startup founders do not understand funding procedures and instinctively fear them.
Moreover, legions of pitch deck service providers propagate a “fairy-tale” approach to the funding process, encouraging founders to beatify their pitch decks to impress investors. Instead of providing quality information, this approach is about style and formatting. The pitch deck is the tip of the funding iceberg. Located below the surface relevant and trustworthy information can help founders to pass the sanity check and due diligence, overcoming the information asymmetry. Both parties need to build a knowledge pyramid depicted in Fig. 1.

Figure1. The data lifecycle in the knowledge pyramid
Data has a lifecycle including three main stages, corresponding to the three levels of the information pyramid: from data to information to knowledge. Technology creates opportunities to manage the data lifecycle, but there are some challenges at each level of the data lifecycle.
Data-Driven Screening vs Gut Feeling
Traditionally, the gut feeling was a preferable decision-making investor’s tool. It is not an effective way of action because of subconscious biases, irrational assumptions, and personal feelings all investors are prone to. Nowadays, a data-driven approach, which uses specific data sets and algorithms, is spreading in the PE industry. This approach allows us to process information with higher levels of accuracy and speed than any investor even with good intuition and experience.
There are three base reasons why more than half of investors continue to rely heavily on gut feeling today:
- Excess of the available data but a lack of quality data. Besides, the difficulties to collect and processing quantitative data contribute to the predominant use of qualitative data. As a result, an essential part of enterprises’ potential remains hidden and untouched.
- Over-reliance on experience when market conditions and business environment are constantly changing making past experiences useless.
- Cognitive biases are psychological features that are common to investors and can influence them to ignore real facts and manage the deal flow with their prejudices. [3] Employing a data-driven approach helps to mitigate some widespread cognitive biases (see Fig. 2).

Figure 2 Overcoming cognitive biases
However, while data-driven mechanisms are more accurate and smarter than humans in theory, they have some essential shortcomings in practice.
AI/ML and Alternative Data
Data-driven approaches seem capable of uncovering pearls and avoiding hidden traps when investors’ common sense fails. This is why employing AI/ML algorithms to predict future startup success has become widespread. In contrast to gut-feeling-based investment decisions, algorithmic ones are unbiased, quick, and can process much bigger amounts of complex data than humans. Furthermore, algorithms can discover profitable deals globally, estimate business opportunities without misjudgments, and weight gains and losses impartially.
All in all, algorithmic sourcing gives quick access to the necessary data, an effective search for opportunities, and easy discovery of red flags. That means cutting down efforts and due diligence expenses, saving investors’ time and money. Due to a lack of quality data to feed AI/ML applications, sophisticated/lead investors can use large volumes of semi-structured alternative data from investment and social platforms such as Angel List, Crunchbase, Pitchbook, and LinkedIn.
It would appear that the issue of AI/ML use is straightforward; however, recent research discovers that underlying data rather than the algorithm itself is the main source of the deviations. In particular, AI/ML algorithms may be trained on datasets that reflect human prejudices and subjectivity. Despite best efforts towards higher objectivity, underlying bias can be built into the AI/ML algorithms themselves. The best practice is to find a good working balance between the algorithmic approach and human engagement.
Merits of a Hybrid Approach
Another point worthy of consideration is that intuition-based decision-making based on long investment experience has proven to be a valuable strategy, especially for early-stage investments. This stage is characterized by a lack of reliable information. To that end, sophisticated investors master to use of soft data such as the team structure, potential customer preferences, etc. They not only employ information that algorithms might not be able to capture but also process this information with the use of effective heuristics to elicit some hidden meanings. As a result, human decisions in some investment situations with a high level of uncertainty demonstrate equal or even better returns than algorithms.
The trends of big data and AI/ML technology allow hope for successful formalisation, but under conditions that include changing stakeholders’ mindsets about data-driven approach, quality of data and its processing, and decision-making process. While the hybrid approach in which artificial and human intelligence (investors and founders) work together in a complementary mode is becoming common, it needs appropriate methods and tools to be more practical.
Traditionally, the equity investment mentality was built on three pillars:
- Strong reliance on historical data patterns within the gut feeling approach, which works well only for revenue-generating enterprises.
- An “apprenticeship model” of startups’ development after funding, when angels sit on the board and advise founders directly.
- The search and screening of prospective startups within restricted well-developed territorial networks, losing lucrative business opportunities that are located outside territories of the Bay Area, New York, etc.
In AI/ML epoch this mentality looks outdated. The AI/ML approach is a promising way to generate SS knowledge from information, but it has inhered limitations. This is why it is necessary to use human- machine methods, employing algorithms to automate the screening process where it makes sense and creates convenience. In some cases where the formalisation is impossible, is reasonable to stimulate founders’ creativity to elicit information.
The PROFIT’s Data-Driven Framework
PROFIT radically transforms the deal sourcing and screening process:
- Gathering data about prospective deals by territory, industry, sector, etc.
- Eliciting meaningful information for analysis in the time and cost-saving mode.
- Uncovering the hidden intangible assets within startups and in the business environment.
- Adding information from alternative sources to improve screening and further due diligence.
To meet the investors’ requirements, the information is:
- Accessible: easily retrieved by investors in a form that is suitable for the screening.
- Identifiable: having identifiers that describe a source, a collection method, and a type of data.
- Interoperable: can be combined with other data sets from different sources for further analysis.
- Reusable: may be processed repeatedly for different purposes.
Creating quality datasets is a challenge. Other challenges are information asymmetry and behavioural/attitudinal matters. The PROFIT.enterprises platform offers effective solutions to the most painful problems.
Sourcing and screening challenges:
- Information asymmetry when investors do not have enough information about the prospects of enterprises’ development or available information of low quality. This information is absent or concealed by the founders.
- Difficult to collect qualitative and quantitative data from different sources with a clear perspective to integrate them. This disadvantage, in turn, reduces the quality of the information analysis and forecasts about possible startup performance.
- Obvious shortcomings in both intuitive investments and algorithmic methods. The former is inefficient due to the limited human capacity to process big volumes of data. Algorithms are not able to capture some information and process it without the use of effective human heuristics.
- Investors’ biases prevent them from objectively assessing business opportunities. They can ignore logic and real facts, relying on their assumptions.
PROFIT’s solutions:
- Employing a “knowledge transfer” method in which comprehensive and quality information is formed step-by-step in the Startup Dossier by founders. Then, founders transfer this curated information to investors, making the sourcing and screening process more efficient.
- Collecting data from three data sources: internal, created inside enterprises; modelling, when information about the business environment is inbuilt in algorithms, and alternative – from open online sources.
- Utilising the hybrid approach in which human expertise and algorithmic solutions complement each other.
PROFIT allows the collecting of raw data inexpensively, aggregating datasets with high accuracy, and stimulating insights to create knowledge bases. People and algorithms have complementary strengths and weaknesses, and each party uses different sources and types of data. Within the hybrid approach, applying heuristics and intuition together with algorithms make it possible to reach reasonable accuracy of results.
The PROFIT platform uses the data-driven Startup Dossier to collect, model, and keep information about each step of the preparation for the funding journey. [4] The information flow on the platform goes to three levels of data transformations, providing useful knowledge for users (startup founders and investors):
1st level – Founders obtain access to the platform, create their profile, bring raw data, and transform it into a structured data set with the use of PROFIT tools.
2nd level – Founders use PROFIT algorithms to reach startups’ fitness for funding.
3rd level – Industry benchmarks, market, and regulation data added to the database to be integrated with information from the 1st and 2nd levels. Curated information about startups becomes available for investors (see Fig. 3).
Data templates in the Dossier allow for standardising formats of the datasets and cleaning data for predictive analytics. In filling the Startup Dossier founders use algorithms when it makes sense. In other cases, they use their creativity to elicit and process information. This data-driven hybrid approach makes the deal sourcing and screening process more adaptable to the changing economic environment.
- The PROFIT system mitigates the most common biases:
- Providing realistic estimation of risks.
- Modelling financials and roadmaps in the united circle of “just-in-time” funding. • Offering exit scenarios, taking into consideration investors’ preferences.
Dossier Workflow

Figure 3 PROFIT’s data flow
The Dossier is the central hub of information to prepare all necessary funding-related information for further presentation to investors, who make screening and further steps of deal flow. Founders work in a self-serve mode, having access to their accounts that allow them to produce, renew, and edit information. This way, they can form pitch decks and other startup promotion materials. Thus, investors can discover startups that can present comprehensive and reliable information for deal sourcing and screening.
Finally, it is important to note that the PROFIT.enterprises platform is significantly different from existing on the market thanks to the unique data diligence design built on the following principles:
- Employing the hybrid approach in which effective algorithms are complemented by human creativity.
- Combining self-assessment and self-learning mechanisms on one platform.
- Providing tools for collecting and processing information, keeping all findings in the Startup Dossier.
- Creating a solid information background for startups to be fit for funding: investors obtain trustworthy, thoroughly curated, and complete information about investment opportunities to
- make well-weighted decisions.
The PROFIT framework allows collecting and keeping startups’ data to develop realistic datasets for ML algorithms and meaningful information for investors’ decision-making.
References
[1] Data-driven VC #1: Why VC is broken and where to start fixing it;
https://www.datadrivenvc.io/p/data-driven-vc-1-why-vc-is-broken
[2] How Venture Capitalists Make Decisions;
https://hbr.org/2021/03/how-venture-capitalists-make-decisions
[3] 23 Investing Biases and How to Avoid Them;
https://www.optimizedportfolio.com/investing-biases/
[4] Introducing Profile Dossiers — Beautiful Reports On Startups, Competitors, And Partners;
About PROFIT.enterprises:
PROFIT.enterprises is a technology platform that is focused on initial sourcing and screening for investments in early-stage startups. The platform kicks startups to get fit for funding and shows investors attractive business opportunities via the discovery and appropriation of intangible assets. As a data intelligence partner, PROFIT.enterprises provides investors with trustworthy information about startups’ prospective profitability and risks, making the deal flow efficient, quick, and convenient.
PROFITTM is a registered Australian trademark No 1949116. All other products and brands mentioned in this document are properties of their respective owners.